CT-based radiomics and clinical characteristics for predicting bone metastasis in lung adenocarcinoma patients
Highlight box
Key findings
• Computed tomography-based clinical radiomics nomogram can be used to predict bone metastasis (BM) in lung adenocarcinoma patients.
What is known and what is new?
• Radiomics features have been confirmed to characterize the heterogeneity of tumors and have been widely used to predict the biological behavior of tumors.
• We developed and validated three models to predict the risk of BM in lung adenocarcinoma patients.
What is the implication, and what should change now?
• Our clinical radiomics nomogram can help clinicians stratify the risk of BM in lung adeno-carcinoma patients, thereby enabling personalized clinical decision making.
Introduction
Lung cancer stands as a leading cause of cancer-related mortality in the present day, with approximately 2.2 million individuals worldwide receiving a diagnosis of this malignancy annually (1,2). Adenocarcinoma emerges as the predominant histological subtype within the spectrum of lung cancer, comprising over 40% of all primary lung cancer cases (3,4). Compared to other subtypes, adenocarcinoma is noted for its heightened propensity for bone metastasis (BM), with an estimated 20–50% of lung adenocarcinoma patients experiencing this occurrence (5,6). Unfortunately, the survival outlook for patients enduring BM is notably bleak compared to those with metastases in the respiratory and nervous systems (7). The median survival duration post-BM diagnosis is a mere 6–10 months (8,9). Additionally, a significant portion of BM patients (approximately 46%) experienced skeletal-related events, such as bone pain, pathologic fractures, spinal cord compression, and hypercalcemia (10,11). Furthermore, there is a lack of effective treatment methods after the occurrence of BM, traditional anti-tumor therapies may have limited efficacy in treating BM (12). Hence, developing a reliable risk stratification tool for BM in lung adenocarcinoma patients is crucial for identifying individuals at high-risk of developing BM at an early stage. By accurately identifying high-risk patients, healthcare providers can implement proactive monitoring strategies and offer timely interventions to improve patient outcomes and quality of life.
Computerized tomography (CT) has been widely used in the diagnosis, staging and efficacy evaluation of lung adenocarcinoma because of its advantages of being non-invasiveness, economy and convenience (13-15).
Traditional CT signs can indicate the aggressiveness of lung adenocarcinoma to some extent. Song and colleagues confirmed that the vascular tumor thrombus of lung adenocarcinoma was associated with solid components and lobulated and calcified features in CT images, while nerve invasion was related to features with bronchial inflation sign in CT images (16). However, these studies frequently rely on conventional subjective assessment signs or rudimentary measurements, such as tumor diameter, to assess disease, while ignoring the differences in biological behavior caused by tumor heterogeneity (17,18).
Radiomics involves extracting extensive and intricate information from medical imaging data in a high-throughput fashion, revealing details beyond human visual perception (19). These quantitative or semi-quantitative data from the images can offer insights into the heterogeneity and genetic traits of the lesions (20-22). In recent years, radiomics has been widely used in the diagnosis, clinical staging, histopathological classification, genetic diagnosis, therapeutic effect evaluation, prognosis prediction of lung adenocarcinoma (23-27). However, there are few studies on radiomics for predicting BM in lung adenocarcinoma patients. Therefore, this study aims to investigate the value of clinical characteristics and CT radiomics in predicting BM in lung adenocarcinoma patients. We present this article in accordance with the TRIPOD reporting checklist (available at https://tlcr.amegroups.com/article/view/10.21037/tlcr-24-38/rc).
Methods
Patients and clinical characteristics
The retrospective study was approved by ethics committee of the Affiliated Hospital of Qingdao University (No. QYFYWZLL28400) and individual consent for this retrospective analysis was waived. The study was conducted in accordance with the Declaration of Helsinki (as revised in 2013).
This study included 1,369 patients with confirmed pathologic lung adenocarcinoma diagnosed at the Affiliated Hospital of Qingdao University from March 2017 to March 2019. Following rigorous selection criteria, a subset of patients was excluded: (I) synchronous BM at baseline examination; (II) with other primary malignant tumors; (III) incomplete clinical and follow-up data or poor CT image quality. Finally, 501 patients were enrolled in the study. Figure 1 illustrates the recruitment process of patients. It is pertinent to highlight that, for the precise quantification of the correlation between BM and potential risk factors, individuals presenting with pre-existing BM at the initial diagnosis of lung adenocarcinoma were intentionally omitted from the study cohort (n=279). Patients were randomly assigned to the training cohort (n=351) and the validation cohort (n=150) in a 7:3 ratio.
Previous studies have identified gender, age, tumor diameter, serum carbohydrate antigen 125 (CA125), alkaline phosphatase (ALP), and degree of differentiation as independent risk factors for BM in lung cancer (28-30). Based on this, we selected clinical factors including general clinical information, histopathological information, and traditional CT features. General clinical information include age, gender, smoking history, stage, serum carcinoembryonic antigen (CEA), CA125, cytokeratin-19-fragment (CYFRA21-1) and ALP within 2 weeks before surgery or puncture. Stage was evaluated by the 8th edition of the International Association for the Study of Lung Cancer (IASLC) staging system (31). The histopathologic information was interpreted by a pathologist with 5 years of experience in pulmonary diseases, included the Ki-67 expression level, and epidermal growth factor receptor (EGFR) gene status. Traditional CT features included primary tumor maximum diameter, location (peripheral/central), shape (regular/irregular), morphology (solid/mixed/ground-glass), edge (clear/vague), lobulation (yes/no), spiculation (yes/no), cavity (yes/no), vacuole (yes/no), air bronchogram (yes/no), pleural traction (yes/no), pleural thickening (yes/no), pleural effusion (yes/no) and vessel convergence (yes/no). Two experienced radiologists, with 5 and 8 years of professional experience, respectively, independently observed the conventional CT features without prior knowledge of BM status. Consensus was reached when both radiologists shared the same opinion, while any discrepancies were resolved through discussion and final agreement with a senior radiologist boasting over 15 years of diagnostic expertise.
CT image acquisition and preprocessing
All patients underwent chest CT scans prior to diagnosis, with scanning parameters outlined in Table S1. To mitigate variances resulting from varied parameters, image standardization was implemented using the PyRadiomics python software plugin. Voxel sizes were resampled to a consistent 3×3×3 mm3 dimension to ensure uniformity (the bin width of the gray value discretization was set to 25) (32).
Follow up
The date of last follow-up was June 30, 2023. The end point of this study was BM identified by bone scanning, positron emission tomography/computed tomography (PET/CT), or biopsy. Patients were followed at least every 6 months for 2 years after diagnosis and at least annually thereafter, with data collected through health-system inquiries and telephone calls.
Clinical model development
Clinical characteristics were screened using univariate and multivariate analyses to identify independent predictors associated with BM, and clinical models were developed based on these independent predictors. Odds ratio (OR) for each factor was used as an estimate of the relative risk with 95% confidence intervals (CIs).
Segmentation and feature extraction
Segmentation of 3D regions of interest (ROI) was performed with the use of ITK-SNAP software (version 3.8.0, www.itksnap.org) (33).
The images were initially segmented by Radiologist 1, following which 60 patients’ CT images were randomly chosen from the entire dataset and re-segmented by Radiologist 2. Inter-class correlation coefficient (ICC) was employed to assess the reproducibility of radiomics features. Features extracted from both segmentations were compared, and only those exhibiting an ICC >0.75 were kept for further analysis (34).
The radiomics features were extracted using the python software plug-in PyRadiomics. A total of 1,130 radiomics features were extracted, including 14 shape features, 18 first-order features, 24 Gray Level Co-occurrence Matrix (GLCM) features, 14 Gray Level Dependence Matrix (GLDM) features, 16 Gray Level Run Length Matrix (GLRLM) features, 16 Gray Level Size Zone Matrix (GLSZM) features, 5 Neighboring Gray Tone Difference Matrix (NGTDM) features, and 1,023 filter and wavelet features. Figure 2 illustrates the flow of the study.
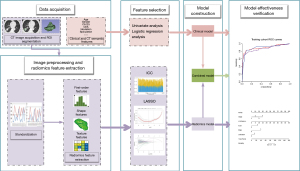
Radiomics feature selection and model development
During model development, the training cohort data underwent dimensionality reduction screening to identify optimal radiomics features for model construction. Subsequently, the validation cohort data was inputted into the developed model for verification, assessing its predictive performance.
To prevent overfitting, a two-step process was employed for dimension reduction of radiomics features in the training cohort. Initially, distinguishing features between the BM and non-BM groups were identified through analysis of variance (ANOVA). Subsequently, the optimal features were chosen using the least absolute shrinkage selection operator (LASSO) algorithm (35). Combining the selected features, the radiomics model was developed, and the radiomics score (Rad-score) was calculated for each patient. The nomogram was then constructed, incorporating radiomics features and independent clinical predictors, assigned individual scores on a 0–100 scale, and summed to determine the overall risk of BM in lung adenocarcinoma patients. Calibration curves were utilized to evaluate the alignment between predicted outcomes and actual observations.
Efficacy evaluation of models
Receiver operating characteristic (ROC) curves were generated, area under the curve (AUC), accuracy, sensitivity and specificity were used to evaluate the efficacy of the three models (clinical model, radiomics model and combined model). The DeLong test was used to compare the performance of the models.
Decision curve analysis (DCA) was use to evaluate the clinical utility of the prediction model by calculating the net gain at different probability thresholds (36).
Statistical analysis
SPSS software (Version 25.0, IBM) was used for univariate analysis (including Chi-squared or Mann-Whitney U test). ANOVA, ICC, LASSO regression analysis, ROC analysis, calibration curve, DCA were performed in R statistical software (Version 4.1.0, https://www.r-project.org). Two-sided P<0.05 were considered to indicate statistical significance.
Results
Clinical characteristics
Among 501 lung adenocarcinoma patients, the median follow-up time was 58 months (range, 2–76 months) and 97 patients (19.36%) experienced BM. The baseline data of the patients are shown in Table 1. There was no significant difference in the distribution of clinical characteristics between the training cohort and validation cohort (Table S2). After univariate and multivariate analysis, 5 independent risk predictors for BM in lung adenocarcinoma patients were determined: CYFRA21-1 (OR =1.211, P=0.002), stage (OR =2.637, P<0.001), Ki-67 (OR =26.444, P=0.006), edge (OR =9.752, P<0.001), and lobulation (OR =4.308, P=0.02). The results of the multivariate analysis of clinical characteristics are shown in Table S3.
Table 1
Characteristics | Training cohort (n=351) | Validation cohort (n=150) | |||||
---|---|---|---|---|---|---|---|
BM (+) | BM (−) | P value | BM (+) | BM (−) | P value | ||
Age, year, median (range) | 59.00 (52.00–65.00) | 61.00 (53.00–66.00) | 0.296 | 60.00 (53.50–63.50) | 61.00 (52.00–65.00) | 0.656 | |
Maximum diameter, mm, median (range) | 35.50 (21.00–45.00) | 20.00 (13.00–30.00) | <0.001 | 34.00 (23.50–43.50) | 21.00 (13.50–28.00) | <0.001 | |
CEA, ng/mL, median (range) | 13.60 (2.98–47.50) | 1.65 (0.93–3.16) | <0.001 | 4.92 (2.19–26.00) | 1.63 (1.02–3.87) | <0.001 | |
CA125, U/mL, median (range) | 21.53 (10.25–75.00) | 10.00 (7.51–15.65) | <0.001 | 14.00 (8.00–67.00) | 11.00 (8.00–14.13) | 0.079 | |
CYFRA21-1, ng/mL, median (range) | 3.99 (2.42–7.96) | 2.27 (1.77–3.17) | <0.001 | 3.53 (2.19–5.09) | 2.32 (1.78–3.16) | 0.002 | |
ALP, U/L, median (range) | 72.50 (62.00–88.00) | 67.00 (56.00–82.00) | 0.039 | 72.50 (62.00–85.00) | 68.00 (55.00–84.00) | 0.317 | |
Gender (male/female) | 39/25 | 117/170 | 0.003 | 10/23 | 49/68 | 0.229 | |
Smoking history (yes/no) | 27/37 | 73/214 | 0.007 | 8/25 | 36/81 | 0.467 | |
Ki-67 (≤5%/>5%) | 1/63 | 149/138 | <0.001 | 5/28 | 62/55 | <0.001 | |
EGFR (mutant/wild) | 41/23 | 163/124 | 0.287 | 22/11 | 61/56 | 0.138 | |
Location (peripheral/central) | 54/10 | 278/9 | <0.001 | 29/4 | 115/2 | 0.028 | |
Shape (regular/irregular) | 12/52 | 77/210 | 0.179 | 8/25 | 23/94 | 0.566 | |
Edge (clear/vague) | 15/49 | 183/104 | <0.001 | 9/24 | 65/52 | 0.004 | |
Lobulation (yes/no) | 55/9 | 156/131 | <0.001 | 25/8 | 70/47 | 0.094 | |
Spiculation (yes/no) | 29/35 | 87/200 | 0.021 | 18/15 | 37/80 | 0.016 | |
Cavity (yes/no) | 1/63 | 6/281 | 0.785 | 5/28 | 3/114 | 0.016 | |
Vacuole (yes/no) | 9/55 | 38/249 | 0.861 | 4/29 | 17/100 | 0.946 | |
Air bronchogram (yes/no) | 25/39 | 52/235 | <0.001 | 15/18 | 27/90 | 0.011 | |
Pleural traction (yes/no) | 49/15 | 138/149 | <0.001 | 28/5 | 65/52 | 0.002 | |
Pleural thickening (yes/no) | 14/50 | 20/267 | <0.001 | 5/28 | 10/107 | 0.430 | |
Pleural effusion (yes/no) | 19/45 | 14//273 | <0.001 | 6/27 | 3/114 | 0.003 | |
Vessel convergence (yes/no) | 18/46 | 39/248 | 0.004 | 7/26 | 28/89 | 0.744 | |
Morphology (solid/mixed/ground-glass) | 60/3/1 | 159/62/66 | <0.001 | 27/3/3 | 66/20/31 | 0.027 | |
Stage (I/II/III/IV) | 7/15/16/26 | 229/22/22/14 | <0.001 | 8/1/12/12 | 89/8/13/7 | <0.001 |
BM, bone metastasis; CEA, carcinoembryonic antigen; CA125, carbohydrate antigen 125; CYFRA21-1, cytokeratin-19-fragments; ALP, alkaline phosphatase; EGFR, epidermal growth factor receptor.
Radiomics feature selection and model construction
There were 1,094 stable features retained, and 735 features were selected by ANOVA, finally, after LASSO analysis, five radiomics features were used to construct the radiomics model. Figure 3 shows the process of radiomics features selection and its corresponding coefficients. In addition, the Rad-score of each patient were calculated, calculation formula is in Appendix 1.
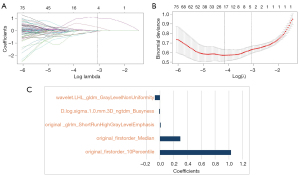
Efficacy and clinical application of the model
The predictive efficacy of the models is shown in Table 2 and the ROC curve (Figure 4) showed that the three prediction models had good performance and could finely predict BM in lung adenocarcinoma patients. In the validation cohort, the AUC of the clinical model, radiomics model and combined model was 0.824 (95% CI: 0.734–0.913), 0.842 (95% CI: 0.754–0.930) and 0.866 (95% CI: 0.786–0.947), respectively. Delong test showed that in the training cohort, the AUC values of the radiomics model and the combined model were statistically different (P=0.03), and the AUC values of the other models were not statistically different, detailed results are provided in Table S4. The integrated nomogram (Figure 5A) incorporating both radiomic and clinical characteristics was developed. The calibration curves (Figure 5B,5C) confirmed close alignment between predicted outcomes and real situation. DCA (Figure 6) showed that the nomogram had a highest net clinical benefit.
Table 2
Model | Training cohort | Validation cohort | |||||||
---|---|---|---|---|---|---|---|---|---|
AUC (95% CI) | Accuracy | Sensitivity | Specificity | AUC (95% CI) | Accuracy | Sensitivity | Specificity | ||
Radiomics model | 0.865 (0.802–0.928) | 0.883 | 0.750 | 0.913 | 0.842 (0.754–0.930) | 0.827 | 0.758 | 0.846 | |
Clinical model | 0.890 (0.837–0.944) | 0.889 | 0.828 | 0.902 | 0.824 (0.734–0.913) | 0.853 | 0.697 | 0.897 | |
Combined model | 0.894 (0.838–0.950) | 0.892 | 0.828 | 0.906 | 0.866 (0.786–0.947) | 0.860 | 0.727 | 0.897 |
AUC, area under the curve; CI, confidence interval.
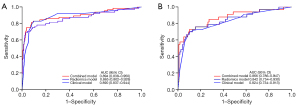
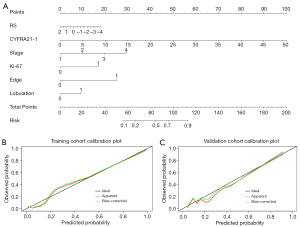
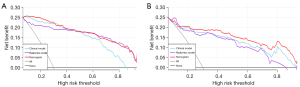
Discussion
In this study, 19.36% of the cohort of patients with lung adenocarcinoma developed BM during the course of their disease. In other studies, the BM rate varied between 20% and 50% (5,6), which may be due to methodological differences between studies. Notably patients who developed BM at the time of diagnosis were excluded from our study population. The endpoint event (i.e., BM) occurs in only about 1/5 of all patients, and how to cope with this data imbalance caused by the epidemiology of the disease or the characteristics of the disease itself is currently controversial. It has been argued that in machine learning research, oversampling for small-sample classification (represented by the SMOTE classical algorithm) can improve model efficacy in some cases, but it has also been pointed out that these algorithms for extended samples tend to lead to overfitting and do not really improve model performance (37-39), in our study, we did not perform sample balancing on the raw data in order to avoid model overfitting problems. Our findings underscore the strong predictive capabilities of three models for forecasting BM in lung adenocarcinoma, with the integrated radiomics and clinical characteristics model exhibiting superior predictive efficacy compared to singular clinical or radiomics models. This model achieved an impressive AUC of 0.894 and 0.866 in the training and validation cohorts, respectively.
Many studies had focused on the risk predictors of BM in NSCLC, Li et al. analyzed the clinical characteristics of 50,581 NSCLC patients based on machine learning, and the results showed that the sex, grade, laterality, histology, T stage, N stage, and chemotherapy were independent risk predictors of BM (40). In a study of risk predictors for distant metastasis (DM) in patients with completely resected lung adenocarcinoma patients, larger tumor size, lymph node metastasis, and vascular lymphatic invasion were considered to be significantly associated with the occurrence of BM (41). In our study, stage, Ki-67, edge and lobulation were thought to be associated with the occurrence of BM, which are basically consistent with the results of previous studies. It is worth to mention that we found that abnormal elevation of CYFRA21-1 was associated with the occurrence of BM, which is consistent with Zhang et al.’s opinion that CYFRA21-1 levels have a stronger correlation with the occurrence of metastasis in patients with lung cancer, especially lung adenocarcinoma (42).
By translating images into quantitative data, radiomics is poised to emerge as a novel tool for depicting tumor heterogeneity and guiding personalized treatment strategies. Previous studies have confirmed the potential value of radiomics in predicting DM of lung adenocarcinoma patients. Coroller et al. delineated that 35 CT-based radiomics attributes exhibited correlations with DM occurrences (43). Furthermore, Peng et al. developed and validated 5 models leveraging clinical and CT radiomics features in a cohort of 253 patients with solid lung adenocarcinoma for predicting DM. Ultimately, their findings highlighted that an integrated model encompassing three-dimensional (3D), two-dimensional (2D) radiomics features alongside clinical characteristics yielded superior predictive efficacy (AUC =0.892) (44). However, the application of radiomics in predicting BM of lung adenocarcinoma has not been reported.
In our study, 5 CT-based radiomics features were found to be associated with BM in lung adenocarcinoma, most (3/5) of which were texture features. Texture features use the distribution characteristics of gray levels in medical images to evaluate the heterogeneity within lesions (45), previous studies have found that texture features of CT images are related to tumor metabolism and staging in NSCLC (46), and have the potential to act as imaging biomarkers for reflecting tumor hypoxia and angiogenesis (47). In a recent study, CT images of 428 patients with clinical stage IA lung adenocarcinoma were analyzed, and the results showed that the texture-based nomogram could predict the pathological aggressiveness of early lung adenocarcinoma with an AUC of 0.849 (48). The study by Sacconi et al. also demonstrated a potential association between CT texture features and EGFR and survival in patients with lung adenocarcinoma (49). Based on the above research, we suggest that these radiomics features representing tumor microenvironment and heterogeneity information may be related to BM in patients with lung adenocarcinoma, and the constructed radiomics prediction model achieved good prediction performance (AUC =0.842 in the validation cohort). In addition, the performance of the combined model by including radiomics and independent clinical risk predictors has been improved in predicting BM in lung adenocarcinoma patients, with an AUC of 0.866 in the validation cohort. This tool helps identify patients at high risk for BM prior to surgery, triggering closer monitoring and necessary interventions by clinicians.
Inevitably, there are some limitations to the study. First, it is a retrospective study, which may lead to selection bias. Second, although radiomics has been shown to be a potential tool for characterizing tumor heterogeneity, there is still a lack of standard radiomics processing procedures for image acquisition and segmentation, feature selection, and model evaluation, and well-designed prospective clinical trials with good quality control are needed. Furthermore, the optimal approach for model development involves the creation of three distinct cohorts for training, testing, and external validation. Regrettably, due to limitations in sample size, our study only implemented training and validation cohorts. Moving forward, efforts will be directed towards expanding the sample size to facilitate the creation of a more robust and scientifically sound model.
Conclusions
In conclusion, the CT-based clinical radiomics nomogram can be used as a non-invasive and quantitative method to help clinicians stratify the risk of BM in patients with lung adenocarcinoma, thereby enabling personalized clinical decision making.
Acknowledgments
Sincere gratitude to all the authors for their invaluable contributions to this study.
Funding: None.
Footnote
Reporting Checklist: The authors have completed the TRIPOD reporting checklist. Available at https://tlcr.amegroups.com/article/view/10.21037/tlcr-24-38/rc
Data Sharing Statement: Available at https://tlcr.amegroups.com/article/view/10.21037/tlcr-24-38/dss
Peer Review File: Available at https://tlcr.amegroups.com/article/view/10.21037/tlcr-24-38/prf
Conflicts of Interest: All authors have completed the ICMJE uniform disclosure form (available at https://tlcr.amegroups.com/article/view/10.21037/tlcr-24-38/coif). The authors have no conflicts of interest to declare.
Ethical Statement: The authors are accountable for all aspects of the work in ensuring that questions related to the accuracy or integrity of any part of the work are appropriately investigated and resolved. The study was conducted in accordance with the Declaration of Helsinki (as revised in 2013). The study was approved by the ethics committee of Affiliated Hospital of Qingdao University (No. QYFYWZLL28400) and individual consent for this retrospective analysis was waived.
Open Access Statement: This is an Open Access article distributed in accordance with the Creative Commons Attribution-NonCommercial-NoDerivs 4.0 International License (CC BY-NC-ND 4.0), which permits the non-commercial replication and distribution of the article with the strict proviso that no changes or edits are made and the original work is properly cited (including links to both the formal publication through the relevant DOI and the license). See: https://creativecommons.org/licenses/by-nc-nd/4.0/.
References
- Siegel RL, Miller KD, Fuchs HE, et al. Cancer statistics, 2022. CA Cancer J Clin 2022;72:7-33. [Crossref] [PubMed]
- Xia C, Dong X, Li H, et al. Cancer statistics in China and United States, 2022: profiles, trends, and determinants. Chin Med J (Engl) 2022;135:584-90. [Crossref] [PubMed]
- Succony L, Rassl DM, Barker AP, et al. Adenocarcinoma spectrum lesions of the lung: Detection, pathology and treatment strategies. Cancer Treat Rev 2021;99:102237. [Crossref] [PubMed]
- Meza R, Meernik C, Jeon J, et al. Lung cancer incidence trends by gender, race and histology in the United States, 1973-2010. PLoS One 2015;10:e0121323. [Crossref] [PubMed]
- Decroisette C, Monnet I, Berard H, et al. Epidemiology and treatment costs of bone metastases from lung cancer: a French prospective, observational, multicenter study (GFPC 0601). J Thorac Oncol 2011;6:576-82. [Crossref] [PubMed]
- Coleman RE. Clinical features of metastatic bone disease and risk of skeletal morbidity. Clin Cancer Res 2006;12:6243s-9s. [Crossref] [PubMed]
- Riihimäki M, Hemminki A, Fallah M, et al. Metastatic sites and survival in lung cancer. Lung Cancer 2014;86:78-84. [Crossref] [PubMed]
- Kuchuk M, Addison CL, Clemons M, et al. Incidence and consequences of bone metastases in lung cancer patients. J Bone Oncol 2013;2:22-9. [Crossref] [PubMed]
- Tie X, Wang J, Wang Y, et al. The prognostic effect of metastasis patterns on overall survival in organ metastatic lung adenocarcinoma. Medicine (Baltimore) 2023;102:e33297. [Crossref] [PubMed]
- Qin A, Zhao S, Miah A, et al. Bone Metastases, Skeletal-Related Events, and Survival in Patients With Metastatic Non-Small Cell Lung Cancer Treated With Immune Checkpoint Inhibitors. J Natl Compr Canc Netw 2021;19:915-21. [Crossref] [PubMed]
- Hakulinen T, Engholm G, Gislum M, et al. Trends in the survival of patients diagnosed with cancers in the respiratory system in the Nordic countries 1964-2003 followed up to the end of 2006. Acta Oncol 2010;49:608-23. [Crossref] [PubMed]
- D'Oronzo S, Coleman R, Brown J, et al. Metastatic bone disease: Pathogenesis and therapeutic options: Up-date on bone metastasis management. J Bone Oncol 2019;15:004-4.
- Zhang Z, Yin F, Kang S, et al. Dual-layer spectral detector CT (SDCT) can improve the detection of mixed ground-glass lung nodules. J Cancer Res Clin Oncol 2023;149:5901-6. [Crossref] [PubMed]
- Li M, Zhang L, Tang W, et al. Identification of epidermal growth factor receptor mutations in pulmonary adenocarcinoma using dual-energy spectral computed tomography. Eur Radiol 2019;29:2989-97. [Crossref] [PubMed]
- Deng L, Tang HZ, Qiang JW, et al. A Nomogram Incorporating Tumor-Related Vessels for Differentiating Adenocarcinoma In Situ from Minimally Invasive and Invasive Adenocarcinoma Appearing as Subsolid Nodules. Acad Radiol 2023;30:928-39. [Crossref] [PubMed]
- Song Y, Chen D, Lian D, et al. Study on the Correlation Between CT Features and Vascular Tumor Thrombus Together With Nerve Invasion in Surgically Resected Lung Adenocarcinoma. Front Surg 2022;9:931568. [Crossref] [PubMed]
- Aerts HJ. The Potential of Radiomic-Based Phenotyping in Precision Medicine: A Review. JAMA Oncol 2016;2:1636-42. [Crossref] [PubMed]
- Constanzo J, Wei L, Tseng HH, et al. Radiomics in precision medicine for lung cancer. Transl Lung Cancer Res 2017;6:635-47. [Crossref] [PubMed]
- McCague C, Ramlee S, Reinius M, et al. Introduction to radiomics for a clinical audience. Clin Radiol 2023;78:83-98. [Crossref] [PubMed]
- Gillies RJ, Kinahan PE, Hricak H. Radiomics: Images Are More than Pictures, They Are Data. Radiology 2016;278:563-77. [Crossref] [PubMed]
- Chen B, Zhang R, Gan Y, et al. Development and clinical application of radiomics in lung cancer. Radiat Oncol 2017;12:154. [Crossref] [PubMed]
- Franceschini D, Cozzi L, De Rose F, et al. A radiomic approach to predicting nodal relapse and disease-specific survival in patients treated with stereotactic body radiation therapy for early-stage non-small cell lung cancer. Strahlenther Onkol 2020;196:922-31. [Crossref] [PubMed]
- Dong Q, Wen Q, Li N, et al. Radiomics combined with clinical features in distinguishing non-calcifying tuberculosis granuloma and lung adenocarcinoma in small pulmonary nodules. PeerJ 2022;10:e14127. [Crossref] [PubMed]
- Huo JW, Luo TY, Diao L, et al. Using combined CT-clinical radiomics models to identify epidermal growth factor receptor mutation subtypes in lung adenocarcinoma. Front Oncol 2022;12:846589. [Crossref] [PubMed]
- Ren J, Chen L, Xu H, et al. A Bi-LSTM and multihead attention-based model incorporating radiomics signatures and radiological features for differentiating the main subtypes of lung adenocarcinoma. Quant Imaging Med Surg 2023;13:4245-56. [Crossref] [PubMed]
- Wang Y, Ding Y, Liu X, et al. Preoperative CT-based radiomics combined with tumour spread through air spaces can accurately predict early recurrence of stage I lung adenocarcinoma: a multicentre retrospective cohort study. Cancer Imaging 2023;23:83. [Crossref] [PubMed]
- Ma X, Xia L, Chen J, et al. Development and validation of a deep learning signature for predicting lymph node metastasis in lung adenocarcinoma: comparison with radiomics signature and clinical-semantic model. Eur Radiol 2023;33:1949-62. [Crossref] [PubMed]
- Zhang C, Mao M, Guo X, et al. Nomogram based on homogeneous and heterogeneous associated factors for predicting bone metastases in patients with different histological types of lung cancer. BMC Cancer 2019;19:238. [Crossref] [PubMed]
- Niu Y, Lin Y, Pang H, et al. Risk factors for bone metastasis in patients with primary lung cancer: A systematic review. Medicine (Baltimore) 2019;98:e14084. [Crossref] [PubMed]
- Zhou Y, Yu QF, Peng AF, et al. The risk factors of bone metastases in patients with lung cancer. Sci Rep 2017;7:8970. [Crossref] [PubMed]
- Travis WD, Asamura H, Bankier AA, et al. The IASLC Lung Cancer Staging Project: Proposals for Coding T Categories for Subsolid Nodules and Assessment of Tumor Size in Part-Solid Tumors in the Forthcoming Eighth Edition of the TNM Classification of Lung Cancer. J Thorac Oncol 2016;11:1204-23.
- Zwanenburg A, Vallières M, Abdalah MA, et al. The Image Biomarker Standardization Initiative: Standardized Quantitative Radiomics for High-Throughput Image-based Phenotyping. Radiology 2020;295:328-38. [Crossref] [PubMed]
- Yushkevich PA, Piven J, Hazlett HC, et al. User-guided 3D active contour segmentation of anatomical structures: significantly improved efficiency and reliability. Neuroimage 2006;31:1116-28. [Crossref] [PubMed]
- Koo TK, Li MY. A Guideline of Selecting and Reporting Intraclass Correlation Coefficients for Reliability Research. J Chiropr Med 2016;15:155-63. [Crossref] [PubMed]
- Alhamzawi R, Ali HTM. The Bayesian adaptive lasso regression. Math Biosci 2018;303:75-82. [Crossref] [PubMed]
- Van Calster B, Wynants L, Verbeek JFM, et al. Reporting and Interpreting Decision Curve Analysis: A Guide for Investigators. Eur Urol 2018;74:796-804. [Crossref] [PubMed]
- Cao Y, Montgomery S, Ottosson J, et al. Deep Learning Neural Networks to Predict Serious Complications After Bariatric Surgery: Analysis of Scandinavian Obesity Surgery Registry Data. JMIR Med Inform 2020;8:e15992. [Crossref] [PubMed]
- Kosolwattana T, Liu C, Hu R, et al. A self-inspected adaptive SMOTE algorithm (SASMOTE) for highly imbalanced data classification in healthcare. BioData Min 2023;16:15. [Crossref] [PubMed]
- Wang S, Dai Y, Shen J, et al. Research on expansion and classification of imbalanced data based on SMOTE algorithm. Sci Rep 2021;11:24039. [Crossref] [PubMed]
- Li MP, Liu WC, Sun BL, et al. Prediction of bone metastasis in non-small cell lung cancer based on machine learning. Front Oncol 2022;12:1054300. [Crossref] [PubMed]
- Hung JJ, Jeng WJ, Wu YC, et al. Factors predicting organ-specific distant metastasis in patients with completely resected lung adenocarcinoma. Oncotarget 2016;7:58261-73. [Crossref] [PubMed]
- Zhang L, Liu D, Li L, et al. The important role of circulating CYFRA21-1 in metastasis diagnosis and prognostic value compared with carcinoembryonic antigen and neuron-specific enolase in lung cancer patients. BMC Cancer 2017;17:96. [Crossref] [PubMed]
- Coroller TP, Grossmann P, Hou Y, et al. CT-based radiomic signature predicts distant metastasis in lung adenocarcinoma. Radiother Oncol 2015;114:345-50. [Crossref] [PubMed]
- Peng Z, Lin Z, He A, et al. Development and Validation of a Comprehensive Model for Predicting Distant Metastasis of Solid Lung Adenocarcinoma: 3D Radiomics, 2D Radiomics and Clinical Features. Cancer Manag Res 2022;14:3437-48. [Crossref] [PubMed]
- Davnall F, Yip CS, Ljungqvist G, et al. Assessment of tumor heterogeneity: an emerging imaging tool for clinical practice? Insights Imaging 2012;3:573-89. [Crossref] [PubMed]
- Ganeshan B, Abaleke S, Young RC, et al. Texture analysis of non-small cell lung cancer on unenhanced computed tomography: initial evidence for a relationship with tumour glucose metabolism and stage. Cancer Imaging 2010;10:137-43. [Crossref] [PubMed]
- Ganeshan B, Goh V, Mandeville HC, et al. Non-small cell lung cancer: histopathologic correlates for texture parameters at CT. Radiology 2013;266:326-36. [Crossref] [PubMed]
- Qiu ZB, Zhang C, Chu XP, et al. Quantifying invasiveness of clinical stage IA lung adenocarcinoma with computed tomography texture features. J Thorac Cardiovasc Surg 2022;163:805-815.e3. [Crossref] [PubMed]
- Sacconi B, Anzidei M, Leonardi A, et al. Analysis of CT features and quantitative texture analysis in patients with lung adenocarcinoma: a correlation with EGFR mutations and survival rates. Clin Radiol 2017;72:443-50. [Crossref] [PubMed]